图书介绍
化学计量学基础pdf电子书版本下载
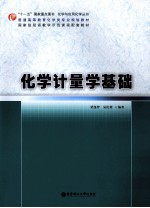
- 梁逸曾,易伦朝编著 著
- 出版社: 上海:华东理工大学出版社
- ISBN:9787562828716
- 出版时间:2010
- 标注页数:196页
- 文件大小:17MB
- 文件页数:209页
- 主题词:化学计量学-高等学校-教材
PDF下载
下载说明
化学计量学基础PDF格式电子书版下载
下载的文件为RAR压缩包。需要使用解压软件进行解压得到PDF格式图书。建议使用BT下载工具Free Download Manager进行下载,简称FDM(免费,没有广告,支持多平台)。本站资源全部打包为BT种子。所以需要使用专业的BT下载软件进行下载。如 BitComet qBittorrent uTorrent等BT下载工具。迅雷目前由于本站不是热门资源。不推荐使用!后期资源热门了。安装了迅雷也可以迅雷进行下载!
(文件页数 要大于 标注页数,上中下等多册电子书除外)
注意:本站所有压缩包均有解压码: 点击下载压缩包解压工具
图书目录
Chapter 1 Introduction and Necessary Fundamental Knowledge of Mathematics 3
1.1 Chemometrics:Definition and Its Brief History 3
1.2 The Relationship between Analytical Chemistry and Chemometries 4
1.3 The Relationship between Chemometrics,Chemoinformatics and Bioinformatic 7
1.4 Necessary Knowledge of Mathematics 9
1.4.1 Vector and Its Calculation 10
1.4.2 Matrix and Its Calculation 19
Chapter 2 Chemical Experiment Design 39
2.1 Introduction 39
2.2 Factorial Design and Its Rational Analysis 41
2.2.1 Computation of Effects Using Sign Tables 44
2.2.2 Normal Plot of Effects and Residuals 45
2.3 Fractional Factorial Design 47
2.4 Orthogonal Design and Orthogonal Array 52
2.4.1 Definition of Orthogonal Design Table 53
2.4.2 Orthogonal Arrays and Their Inter-effect Tables 54
2.4.3 Linear Graphs of Orthogonal Array and Its Applications 55
2.5 Uniform Experimental Design and Uniform Design Table 55
2.5.1 Uniform Design Table and Its Construction 56
2.5.2 Uniformity Criterion and Accessory Tables for Uniform Design 59
2.5.3 Uniform Design for Pseudo-level 60
2.5.4 An Example for Optimization of Eleetropherotic Separation Using Uniform Design 61
2.6 D-Optimal Experiment Design 65
2.7 Optimization Based on Simplex and Experiment Design 68
2.7.1 Constructing an Initial Simplex to Start the Experiment Design 69
2.7.2 Simplex Searching and Optimization 70
Chapter 3 Processing of Analytic Signals 77
3.1 Smoothing Methods of Analytical Signals 77
3.1.1 Moving-Window Average Smoothing Method 77
3.1.2 Savitsky-Golay Filter 77
3.2 Derivative Methods of Analytical Signals 83
3.2.1 Simple Difference Method 83
3.2.2 Moving-Window Polynomial Least-Squares Fitting Method 84
3.3 Background Correction Method of Analytical Signals 89
3.3.1 Penalized Least Squares Algorithm 89
3.3.2 Adaptive Iteratively Reweighted Procedure 90
3.3.3 Some Examples for Correcting the Baseline from Different Instruments 92
3.4 Transformation Methods of Analytical Signals 94
3.4.1 Physical Meaning of the Convolution Algorithm 94
3.4.2 Multichannel Advantage in Spectroscopy and Hadamard Transformation 96
3.4.3 Fourier Transformation 99
Appendix 1:A Matlab Program for Smoothing the Analytical Signals 108
Appendix 2:A Matlab Program for Demonstration of FT Applied to Smoothing 112
Chapter 4 Multivariate Calibration and Multivariate Resolution 116
4.1 Multivariate Calibration Methods for White Analytical Systems 116
4.1.1 Direct Calibration Methods 116
4.1.2 Indirect Calibration Methods 121
4.2 Multivariate Calibration Methods for Grey Analytical Systems 126
4.2.1 Veetoral Calibration Methods 127
4.2.2 Matrix Calibration Methods 127
4.3 Multivariate Resolution Methods for Black Analytical Systems 129
4.3.1 Self-modeling Curve Resolution Method 131
4.3.2 Iterative Target Transformation Factor Analysis 134
4.3.3 Evolving Factor Analysis and Related Methods 137
4.3.4 Window Factor Analysis 141
4.3.5 Heuristic Evolving Latent Projections 145
4.3.6 Subwindow Factor Analysis 152
4.4 Multivariate Calibration Methods for Generalized Grey Analytical Systems 154
4.4.1 Principal Component Regression(PCR) 156
4.4.2 Partial Least Squares(PLS) 157
4.4.3 Leave-one-out Cross-validation 159
Chapter 5 Pattern Recognition and Pattern Analysis for Chemical Analytical Data5.1 Introduction 169
5.1.1 Chemieal Pattern Space 169
5.1.2 Distance in Pattern Space and Measures of Similarity 171
5.1.3 Feature Extraction Methods 173
5.1.4 Pretreatment Methods for Pattern Recognition 173
5.2 Supervised Pattern Recognition Methods:Discriminant Analysis Methods 174
5.2.1 Discrimination Method Based on Euclidean Distance 175
5.2.2 Discrimination Method Based on Mahalanobis Distance 175
5.2.3 Linear Learning Machine 176
5.2.4 k-Nearest Neighbors Discrimination Method 177
5.3 Unsupervised Pattern Recognition Methods:Clustering Analysis Methods 179
5.3.1 Minimum Spanning Tree Method 179
5.3.2 k-means Clustering Method 181
5.4 Visual Dimensional Reduction Based on Latent Proiections 183
5.4.1 Proj ection Discrimination Method Based on Principal Component Analysis 183
5.4.2 SMICA Method Based on Principal Component Analysis 186
5.4.3 Classification Method Based on Partial Least Squares 193